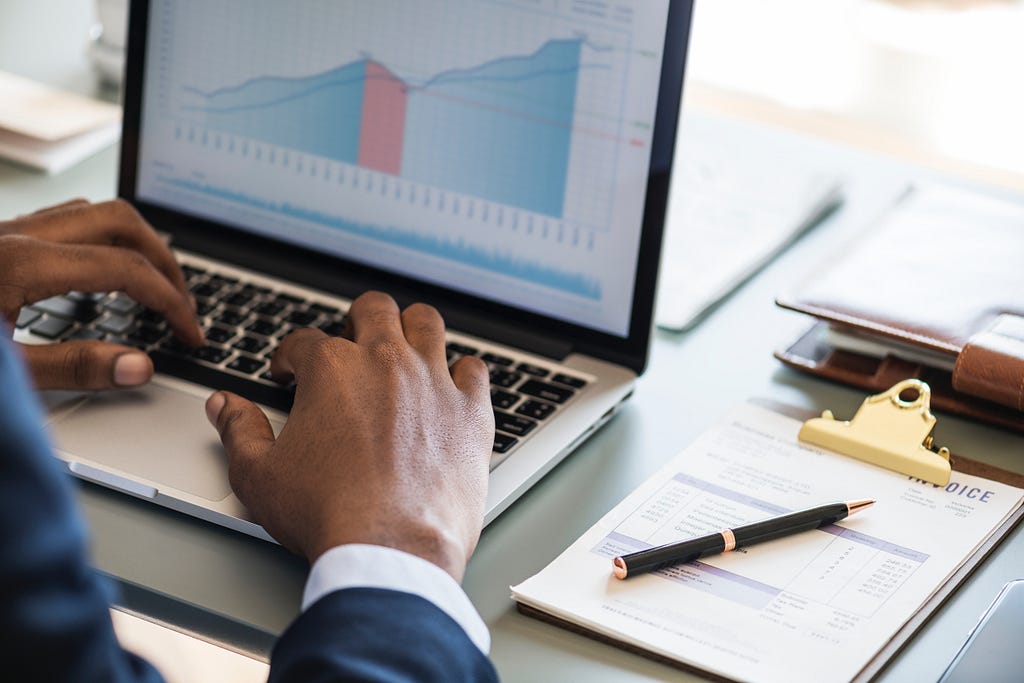
“Turn your dreams into reality with a personal loan,” reads the website of a major bank with a presence in several developing countries. Two of the requirements to get the loan? Be a salaried employee and have a net monthly income of no less than $200.
Yet according to the 2016 Poverty and Shared Prosperity Report by the World Bank, 10 percent of people live on less than $1.90 a day. And half of world’s extreme poor — amounting to 389 million people — live in Sub-Saharan Africa. This dream-come-true loan product locks out most people in developing countries.
But where traditional finance is failing in the developing world, new and innovative technologies — which rely heavily on AI — are poised to transform personal finance for millions.
Take microlending, for instance. Leveraging the peer-to-peer economy, microloans are small loans issued by individuals who each contribute some portion of the total amount. They are often given to people in developing countries who don’t have access to traditional financing and want to start businesses.
Microlending mobile applications like Tala and Branch are in very high demand in the developing world. They collect a range of data about applicants, including biographical information, the number of people they contact daily, the size of their networks and support systems, and their habits. They then use machine learning algorithms (such as regression trees or support vector machines) to train credit scoring models to determine how much to lend.
Tala, present in Kenya, Tanzania and the Philippines, has already disbursed over 5.6 million loans to over a million customers ranging from $10 to $300. Earlier this year, they raised $65 million to expand to Mexico and India.
These microlenders come with a wealth of advantages, including speed and accessibility. Loans can be disbursed almost instantly. Compared to the requirements laid out by banks, microlenders present a much lower barrier to entry, providing a line of credit to individuals who previously would have been unable to scale their small businesses.
Twiga Foods, a business-to-business food distribution company, has introduced a blockchain-based micro-financing product piloted by a couple hundred kiosk owners in Kenya. The company is leveraging blockchain and machine learning to calculate credit scores and disburse microloans.
The loans, which are disbursed through mobile phones, are used as working capital by the kiosk owners, which enables them to purchase and sell higher volumes of food per day. They make an order to Twiga Foods and receive a text message with loan options to pay for the order. In the pilot, order sizes saw an increase of 30 percent, with each retailer’s profits increasing by 6 percent — all with an average loan of about $30.
Despite the benefits of a streamlined and instantaneous loan process, there are also significant challenges. For starters, the loan applications have very high interest rates with equally high penalties for late loan repayment. Lenders justify this by pointing to the riskiness of the investment.

A much higher price paid — one that is not always apparent — is the personal data these individuals are giving away. These products collect everything from biographical data to employment histories to mobile money transactions, and many consumers are unaware of the possible ramifications, including identity theft and financial fraud.
Beyond the dangers of losing control of one’s personal data, the aggregated data of millions of individuals may pose an even greater risk for society, especially in developing markets, where there are few data protection laws and little regulation. Machine learning models automate decision making in scenarios as mundane as targeted advertising, but they could also lead to more exclusionary practices like filtering out female applicants for a technology role. Practitioners as well as policy makers need to collaborate to create frameworks and regulations that address potential biases and ensure that AI doesn’t widen existing socio-economic gaps.
“Beyond the dangers of losing control of one’s personal data, the aggregated data of millions of individuals may pose an even greater risk for society…”
In the case of peer-to-peer lending, AI is widely marketed positively as a way to empower those at the bottom of the pyramid to raise their living standards. At best, it can help individuals grow their small businesses and meaningfully work their way toward financial stability. But giving these populations access to multiple lines of credit without offering financial education also has a flip side: It is not uncommon to find individuals in developing markets with outstanding repayments on more than one of these applications. Individuals could easily find themselves in growing debt, taking on additional loans to repay existing ones.
It is also apparent that these applications are owned by those who have traditionally been at the top of the pyramid. Though their biggest markets are the developing world, Branch has its headquarters in San Francisco while Tala is based in Santa Monica.
Artificial intelligence is poised to permeate and disrupt many different industries. But in order for the overall effects to be truly beneficial for everyone, particularly in the developing world, individuals need to better understand its power; communities need to be empowered with technical knowledge to build their own solutions to their problems; and multidisciplinary teams of practitioners and policy makers need to collaborate to interrogate and mitigate the potential negative consequences.
Traditional Finance is Failing the World’s Poor. Can AI Help? was originally published in Ada-AI on Medium, where people are continuing the conversation by highlighting and responding to this story.